Phytoplankton
The research group has been dedicated to studying the presence of harmful phytoplankton in freshwater reservoirs, which can compromise water quality. Detecting the presence of specific harmful phytoplankton species is crucial for predicting and addressing harmful algal blooms (HAB). To delve into this critical matter, the research group has created a unique phytoplankton dataset, leveraging multi-specimen microscopy imaging. We aim to effectively detect and classify the various species of phytoplankton present in this dataset. For this task, we have tested both classical methods and deep learning approaches.
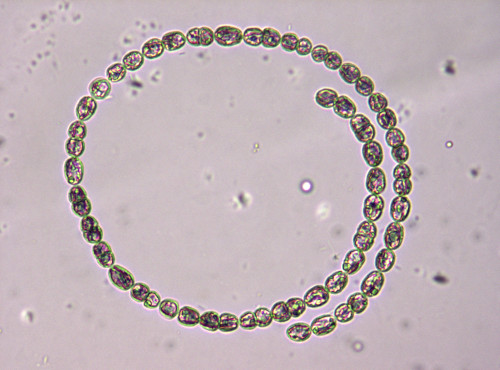
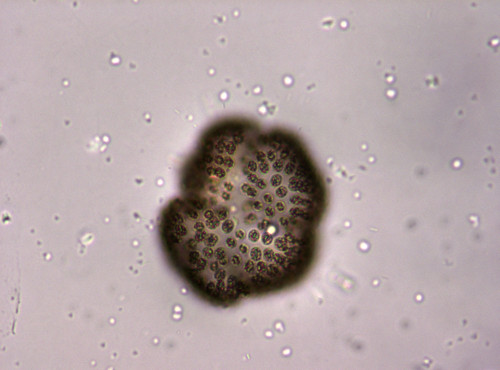
Phytoplankton are microscopic, photosynthetic organisms that inhabit aquatic environments, ranging from oceans to freshwater bodies. As photosynthetic organisms, they harness sunlight to synthesize organic compounds, thus forming the base of aquatic food webs. These microscopic organisms are vital for regulating Earth's atmosphere by sequestering carbon dioxide and producing oxygen through photosynthesis. Their ecological significance extends to influencing climate, nutrient cycling, and supporting diverse aquatic ecosystems. Phytoplankton are one of the main components of the heterogeneous algae group, consisting of various microscopic organisms, including diatoms, dinoflagellates, green algae, cyanobacteria (sometimes called blue-green algae), and other single-celled organisms.
The proliferation of toxic species of phytoplankton, such as certain toxic species of cyanobacteria, poses a significant threat to water quality. In rivers and reservoirs, used for water supply, these harmful algal blooms (HAB) can have a detrimental impact on human health. Furthermore, due to the effects of climate change like alterations in nutrient availability and distribution, HABs are expected to increase in severeness and frequency in the coming years. Currently, experts mostly rely on rudimentary methods for monitoring phytoplankton populations, involving manual sampling and analysis of water. This process entails identifying dangerous species and measuring their biologic volume to compare them to governmental guidelines.
Analysing a large number of samples to obtain a representative measure is a time-consuming and laborious task, further exacerbated by the need for regular monitoring of each separate water source. Moreover, taxonomic identification requires extensive expertise and training for personnel involved. As a result, the entire process is susceptible to subjectivity, potentially affecting its overall accuracy and quality.
In response to these challenges, the complete or partial automation of these tasks emerges as a desirable course of action. Notably, such automation promises to not only reduce the burden on experts but also reduce subjective factors, which threaten the accuracy of the monitoring process.
In order to address these issues, we have created methodologies using firstly classical methods and, then, state-of-the-art deep learning approaches. The first versions of our detection and classification pipeline involved detection using a classical thresholding approach. Then, a domain-specific algorithm was able to locate and fuse nearby blobs belonging to the same specimen through a Delaunay triangulation. Finally, these detected specimens are classified into their different species, using bag of words with handcrafted features such as Gabor filters. This pipeline was later improved adding novel deep features from pre-trained networks (i.e. ResNet trained on ImageNet) in combination with the previously mentioned handcrafted features. This significantly improved the classification results.
In light of the advancements in deep learning methodologies, we have created a novel pipeline based exclusively in deep learning techniques, in order to improve the obtained results further. We tested two state-of-the-art object detectors, Fast R-CNN and RetinaNet. These methods provide notable improvements over the previous classic or hybrid approaches. Firstly, as deep learning directly learns from the data, it eliminates the costly feature tunning process associated to classical methods, known as feature engineering. More importantly, this novel approach significantly improves the results of the previous methods, especially in the detection task.
FMPD: Freshwater Microscopy Phytoplankton Dataset
FMPD (Freshwater Microscopy Phytoplankton Dataset) is a set of multi-specimen microscopy images of freshwater phytoplankton. These images have been captured with fixed settings, equal for each image, including illumination, focal point and magnification. The dataset contains 293 images from water sampled at lake of Doniños (Ferrol, Galicia, Spain) (UTM 555593 X, 4815672 Y; Datum ETRS89) on multiple visits throughout the year. This ensures seasonal representability.
The phytoplankton sample was concentrated by filtering volume of 0.5 L through GF/F glass fiber filters and was then resuspended in 50 mL. Phytoplankton samples were preserved using 5% (v/v) glutaraldehyde, because it is efficient at preserving both cellular structures and pigment. The fixed sample was stored in the dark at constant temperature (10ºC) until analysis. The phytoplankton sample was homogenised for 2 min prior to microscopic examination. In addition, the sample was subjected to vacuum for one minute to break the vacuoles of some cyanobacterial taxa and prevent them from floating. Aliquots of the phytoplankton sample with a total volume of 1 mL were examined under light microscopy using a Nikon Eclipse E600 equipped with an E-Plan 10× objective (N.A. 0.25). Light microscopy images were taken with an AxioCam ICc5 Zeiss digital camera, maintaining the same illumination and focus throughout the image acquisition process and following regular transects until the entire surface of the sample was covered.
The FMPD can be downloaded from here. Please send an email to:Contact email and you will receive an authentication password to access the dataset. This is intended for statistical purposes only, no private data or fee is required. Additionally, the dataset is also hosted on Zenodo for ease of downloading. The data is released for non-comercial academic or research purposes only, subject to attribution through citation of the following papers:
- J. Figueroa, D. Rivas-Villar, J. Rouco, J. Novo, "Phytoplankton detection and recognition in freshwater digital microscopy images using deep learning object detectors", Heliyon, 2024.
- D. Rivas-Villar, J. Rouco, M. G. Penedo, R. Carballeira, J. Novo, "Fully automatic detection and classification of phytoplankton specimens in digital microscopy images", Computer Methods and Programs in Biomedicine, 200, 105923, 2021
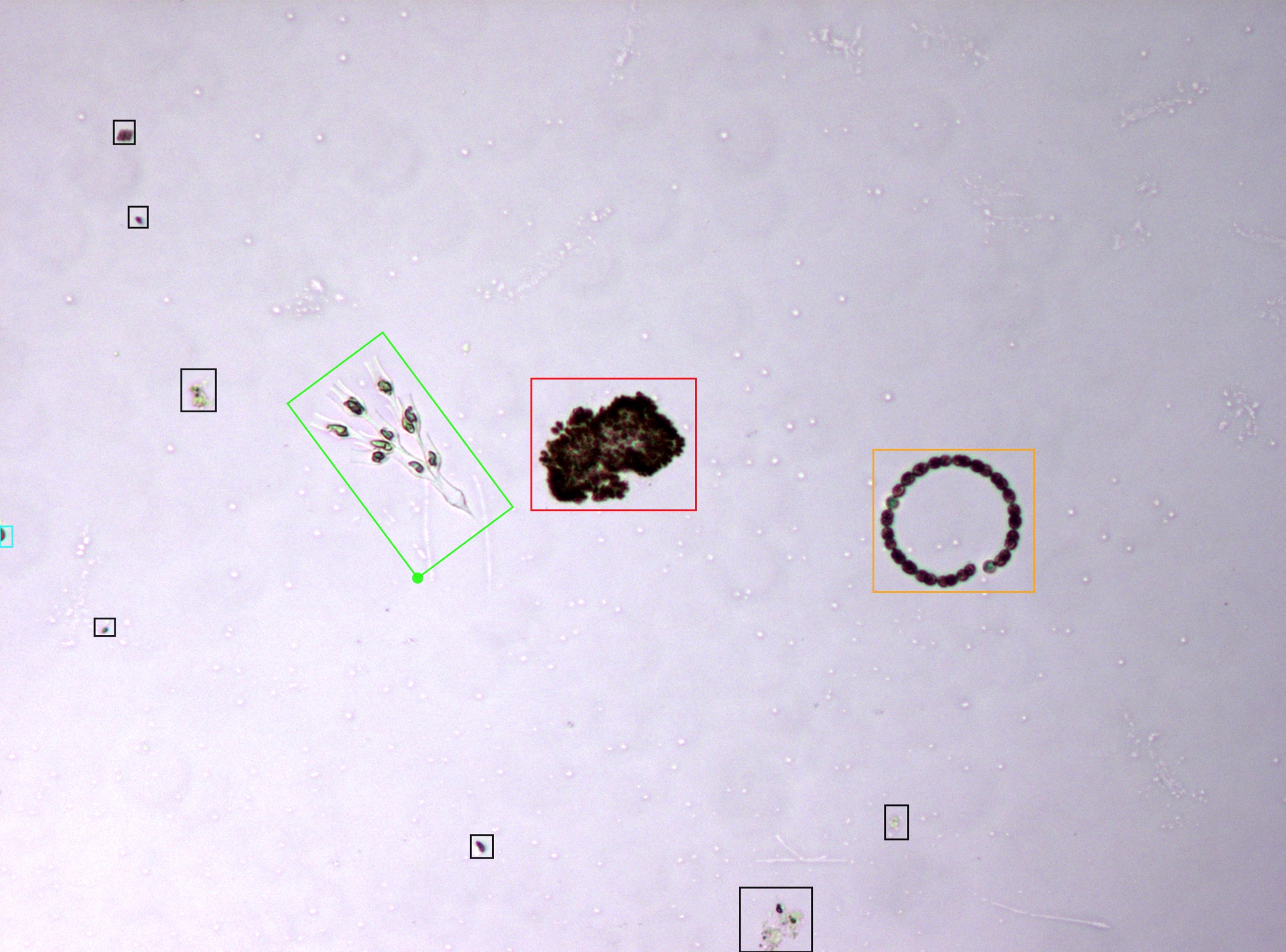
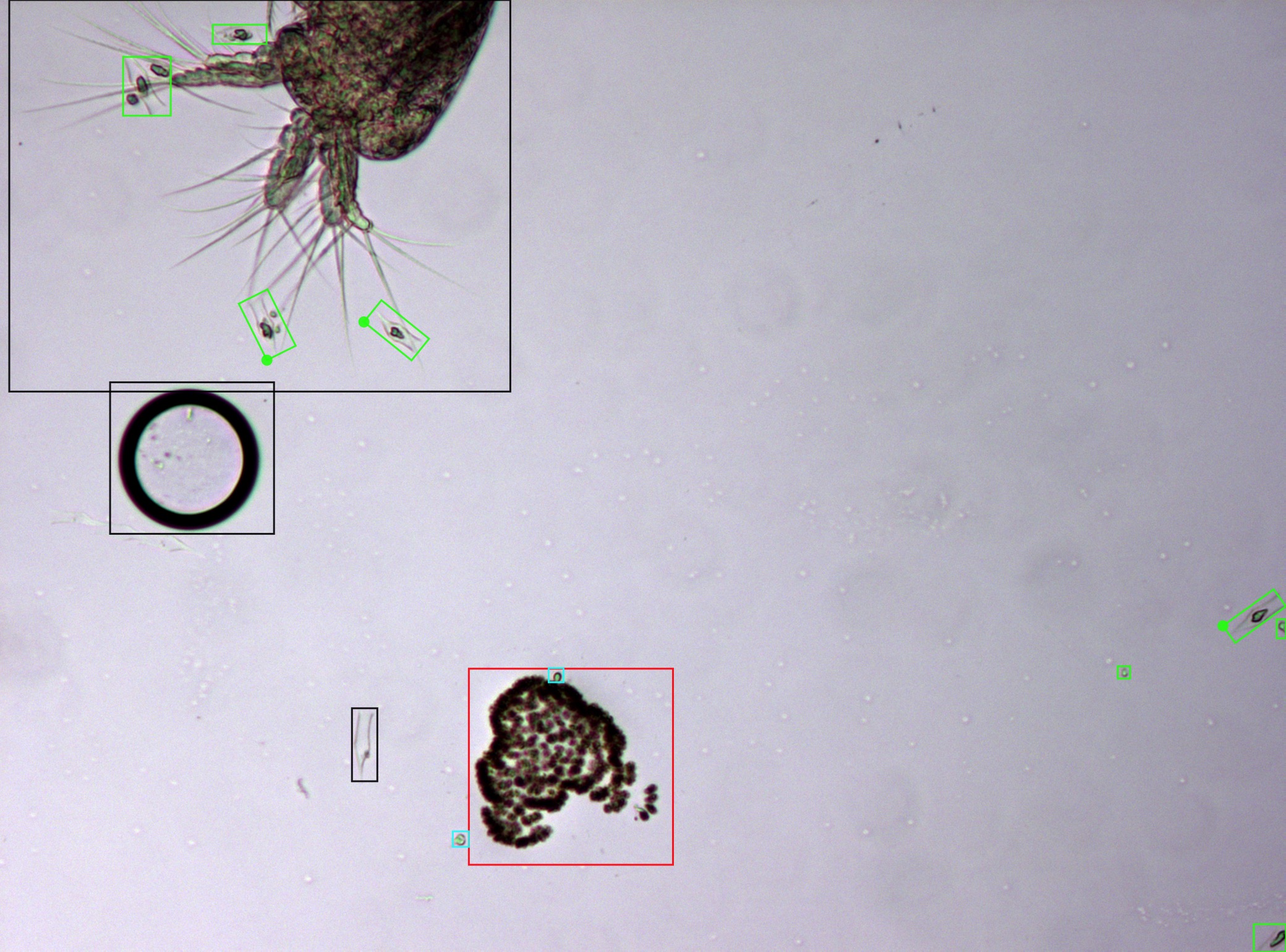
Main publications
-
,
,
,
,
"Phytoplankton detection and recognition in freshwater digital microscopy
images using deep learning object detectors",
Heliyon,
2024.
[Abstract] [PDF]
-
,
,
,
,
"Deep Features-based approaches for Phytoplankton Classification in Microscopy Images",
Lecture Notes in Computer Science: Computer Aided Systems Theory, Revised Selected Papers, EUROCAST 2022,
Las Palmas de Gran Canaria, Spain,
2023.
[PDF] [+Info.] -
,
,
,
,
,
"Fully automatic detection and classification of phytoplankton specimens in digital microscopy images",
Computer Methods and Programs in Biomedicine,
200,
105923,
2021.
[Abstract] [PDF]
-
,
,
,
,
,
"Automatic Detection of Fresh-water Phytoplankton Specimens in Conventional Microscopy Images",
Sensors,
20,
2020.
[Abstract] [PDF]